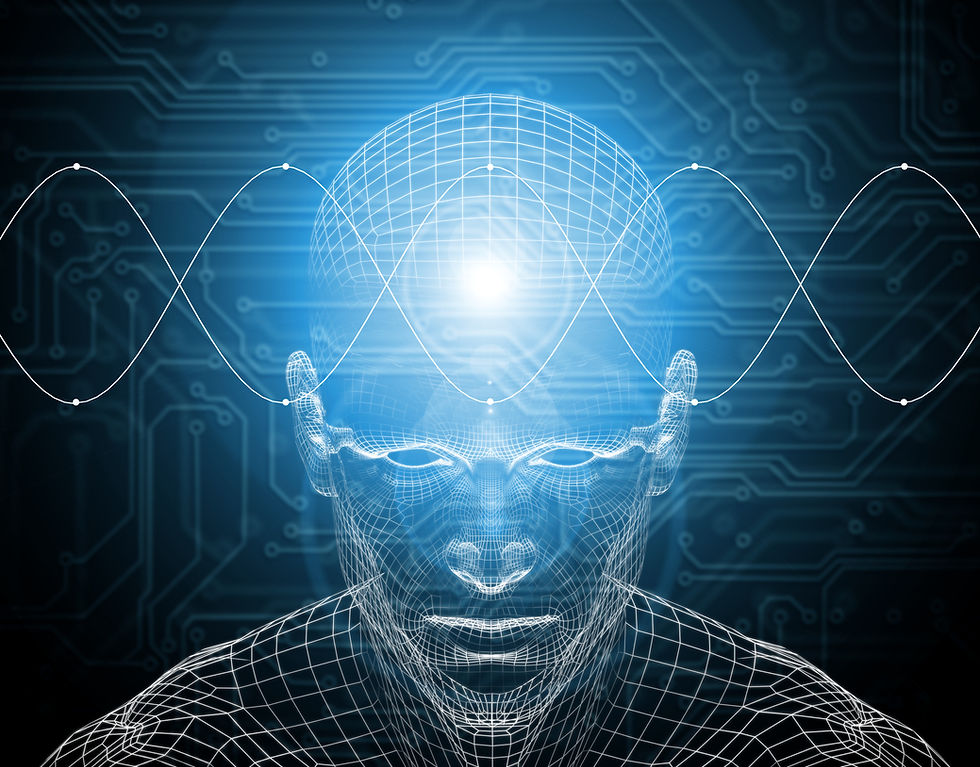
In the rapidly evolving landscape of artificial intelligence (AI), the issue of bias has emerged as a critical concern. As AI systems increasingly shape decision-making processes across various domains, understanding and mitigating bias is essential to ensure fairness and equity. In this article, we'll explore the nuances of AI bias, from its roots in implicit bias within data to its profound impact on fairness and equity, and the challenges inherent in its mitigation.
Implicit Bias in Data: At the core of AI bias lies the data on which algorithms are trained. Unfortunately, this data often reflects the biases and prejudices present in society. Known as implicit bias, these biases can be based on factors such as race, gender, or socioeconomic status. When AI algorithms learn from biased data, they may inadvertently perpetuate or amplify these biases in their decision-making processes. Recognizing and addressing implicit bias in data is crucial for promoting fairness and equity in AI-driven systems.
Impact on Fairness and Equity: The implications of AI bias extend far beyond the digital realm, with real-world consequences for fairness and equity. Biased algorithms can lead to discriminatory outcomes in various domains, including hiring, lending, and criminal justice. For example, biased facial recognition systems may disproportionately misidentify individuals from certain demographic groups, leading to erroneous arrests or surveillance. Addressing AI bias is essential for upholding principles of fairness and equity and safeguarding against discriminatory practices in algorithmic decision-making.
Challenges in Mitigation: Mitigating AI bias presents significant challenges due to the complexity of algorithms and the inherent biases present in data. While various techniques, such as bias detection algorithms and fairness-aware machine learning, have been developed to address bias, eliminating bias entirely remains a formidable task. It requires interdisciplinary collaboration, transparency, and ongoing efforts to identify and mitigate bias throughout the AI development lifecycle. Additionally, the rapid pace of technological advancement and the proliferation of AI systems pose challenges in ensuring that bias mitigation strategies remain effective and up-to-date.
In conclusion, understanding and mitigating AI bias is a multifaceted endeavor that requires concerted efforts from researchers, developers, policymakers, and other stakeholders. By recognizing the presence of implicit bias in data, acknowledging the impact of bias on fairness and equity, and addressing the challenges inherent in bias mitigation, we can work towards building AI systems that are fair, transparent, and equitable. Together, let's navigate the complexities of AI bias and strive towards a more just and inclusive digital future.
Comments